DAIDNet
A Lightweight Domain Aware Intrusion Detection Network for Automated Detection of Network Penetrations
Intrusion detection and prevention has been an area of active research in the use of machine learning for cyber security practices. Artificial Neural Networks (ANN) are one of the best-known approaches when it comes to accurately classifying intrusions into attack classes or benign profiles but they are resource-intensive. A server is typically associated with large a amount of high-frequency data. In such a condition, deploying ANN for this purpose can cause a significant overhead and delays in the delivery of packets to their intended destination. Furthermore, existing deep learning approaches do not address the similarity between different attack classes, the information regarding which can be used to select the defence strategies.
DAIDNet utilizes the information contained by the domain of classes extracted from packet distributions to make better predictions. Results show that DAIDNet achieves better accuracy while being significantly smaller in size than a baseline ANN model. DAIDNet achieves validation accuracy of 99.66% and 99.98% on the NSL-KDD and CICIDS-2018 datasets, respectively which correspond to SOTA or near-SOTA.
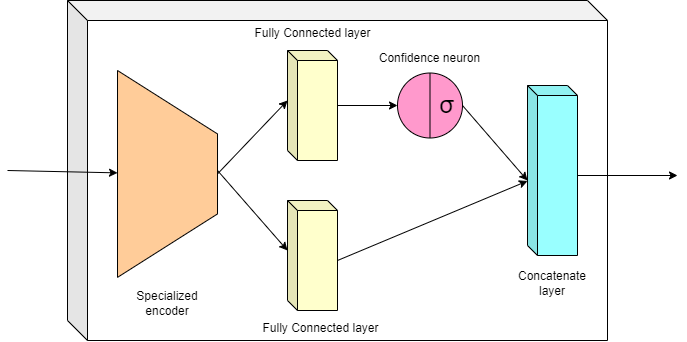
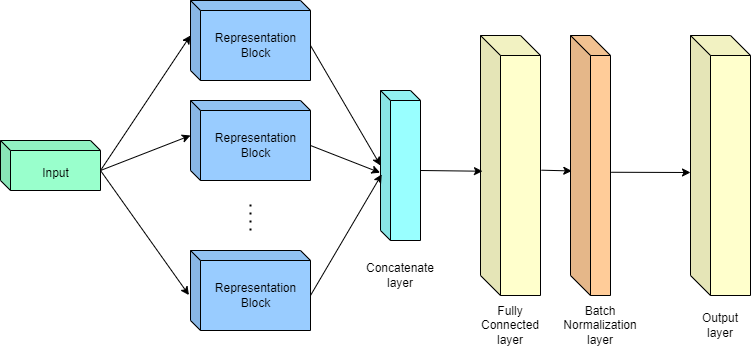
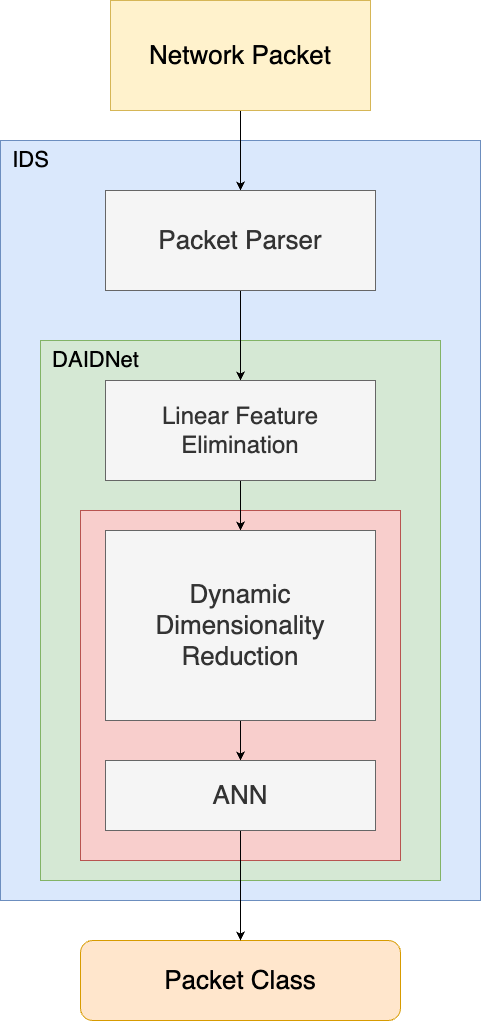
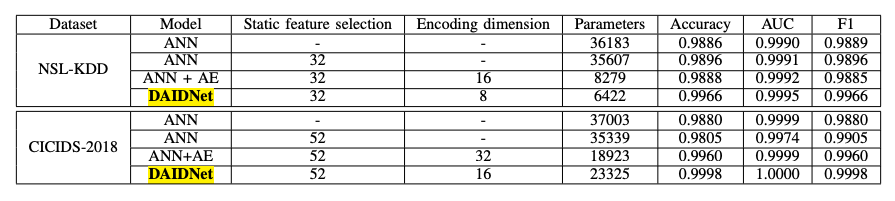